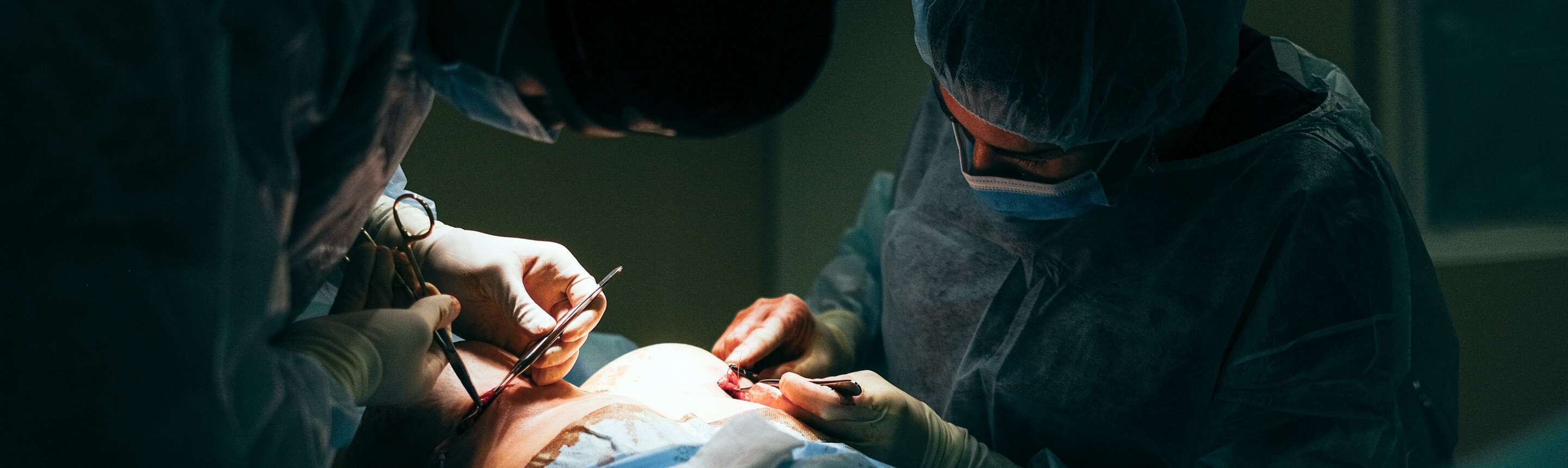
Safety and quality control of algorithmic systems in clinical decision making
Partners: Delft University of Technology & Utrecht University
Type: PhD
Duration: 2023—2026
While medical hospitals have advanced quality and safety controls and assessments for technological tools, these cannot be straightforwardly applied to algorithmic decision systems, due to the complex nature of how these integrate in a clinical treatment or research context. This means emergent safety risks are not yet understood nor appropriately anticipated, and challenges emerge around assigning responsibility to developers versus (quality) management versus clinical personnel. In addition, new regulatory requirements will emerge to ensure effective human oversight. This PhD project will research current quality and safety control practices in academic hospitals, map emergent risks of ADS applications, identify emergent regulatory requirements, and draw lessons from the history of system safety and systems engineering to propose effective paradigms for ensuring quality and safety of ADS in clinical contexts, and to inform regulatory initiatives.